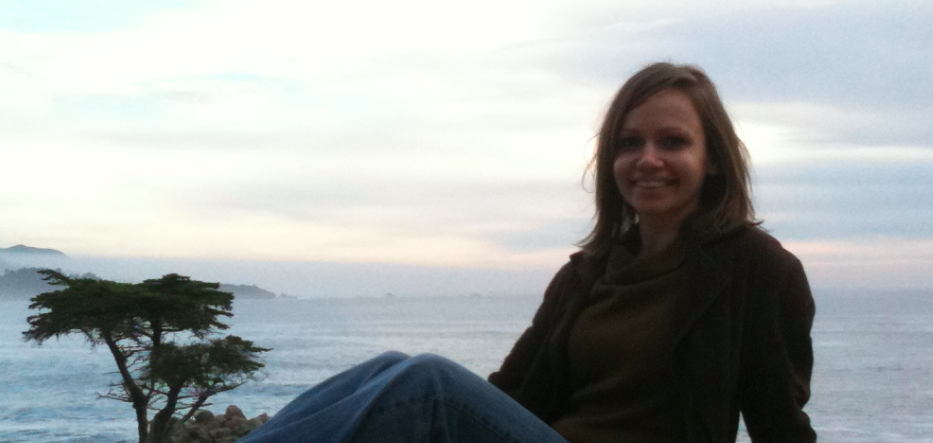
email: | dasta@stat.osu.edu |
office: | 427 Cockins Hall |
Research
I'm generally interested in statistics that either reveals or depends upon interesting geometry in the data.
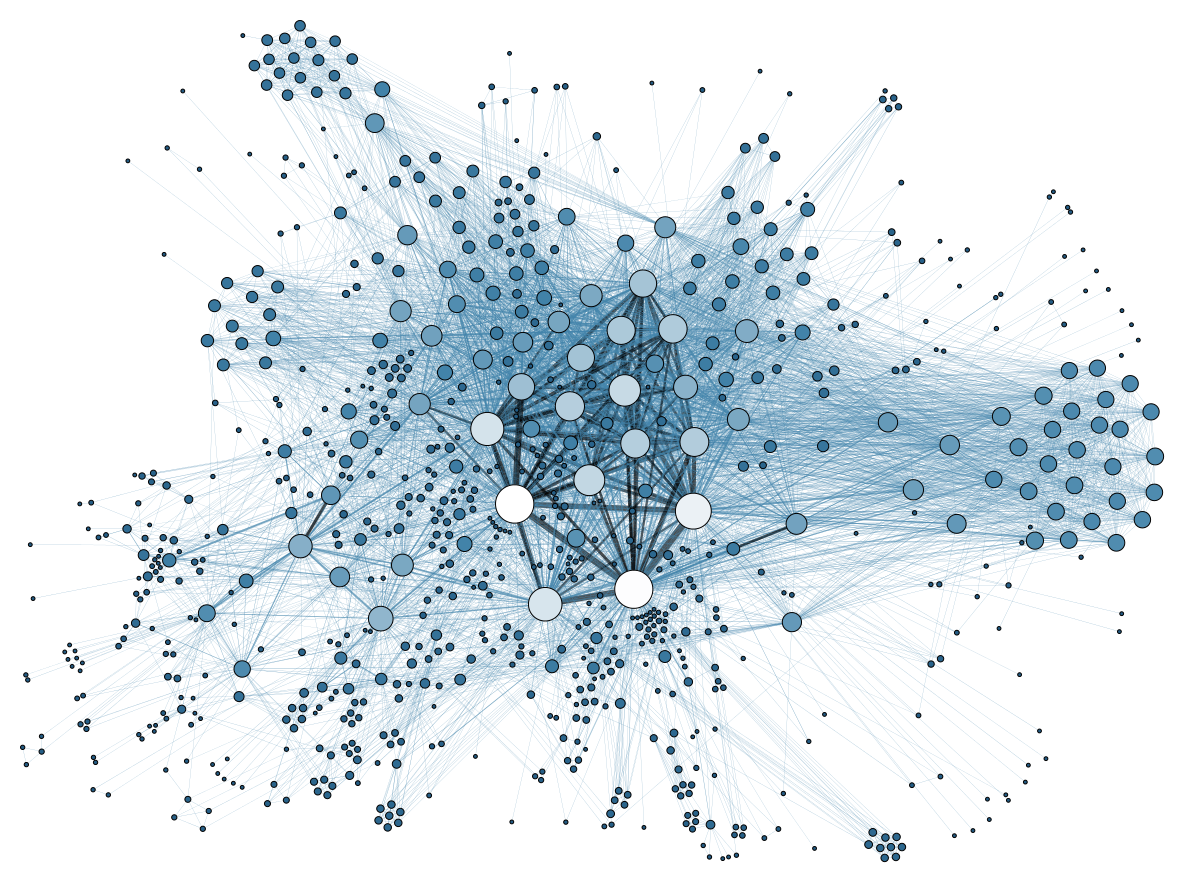
Network Inference
Network inference is about inferring a distribution of random graphs (networks) from sample graphs (networks). This is useful, for example, in assessing whether changes in networks over time, environment, or other conditions are significant or mere fluctuations. It is often natural to assume that the networks of interest are finite approximations of some interesting space. I am interested in the interplay between the geometry of the latent space (e.g. curvature) and the problems of network inference (e.g. estimator consistency).
Network inference is about inferring a distribution of random graphs (networks) from sample graphs (networks). This is useful, for example, in assessing whether changes in networks over time, environment, or other conditions are significant or mere fluctuations. It is often natural to assume that the networks of interest are finite approximations of some interesting space. I am interested in the interplay between the geometry of the latent space (e.g. curvature) and the problems of network inference (e.g. estimator consistency).
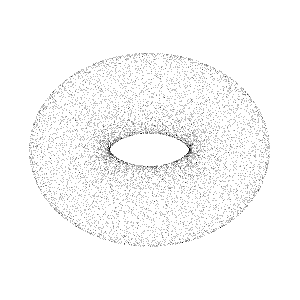
Estimation on Curved Spaces
Data and their generative models are usually parametrized as collections of numbers, but both are often more naturally regarded as points in a non-Euclidean space. Some examples of such data are directional headings (the space SO(3) of 3x3 special orthogonal matrices), distortions in the spacetime continuum (symmetric positive definite matrices), or latent positions of large-scale networks (hyperbolic spaces). In all such examples, Euclidean distances do not reflect the true distance between the data, regarded as points in a true latent space. Similarly, parametric families of generative models are often studied as geometric spaces where the distance between two such generative models is their Fisher distance. I am interested in extending standard constructions and results regarding Euclidean statistics, such as kernel density estimation and maximum likelihood estimation, for the non-Euclidean setting.
Data and their generative models are usually parametrized as collections of numbers, but both are often more naturally regarded as points in a non-Euclidean space. Some examples of such data are directional headings (the space SO(3) of 3x3 special orthogonal matrices), distortions in the spacetime continuum (symmetric positive definite matrices), or latent positions of large-scale networks (hyperbolic spaces). In all such examples, Euclidean distances do not reflect the true distance between the data, regarded as points in a true latent space. Similarly, parametric families of generative models are often studied as geometric spaces where the distance between two such generative models is their Fisher distance. I am interested in extending standard constructions and results regarding Euclidean statistics, such as kernel density estimation and maximum likelihood estimation, for the non-Euclidean setting.
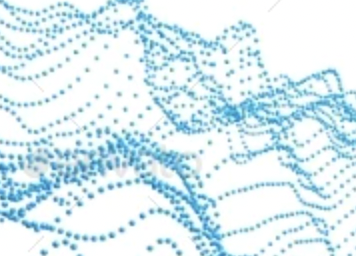
Manifold Learning
Often, the space that the data is sampled from itself is unknown. For example, in computer graphics and machine vision, that space might literally be a geometric object one is trying to learn from imaging data. In another example, in social networks, that space might represent covariates for users with unknown correlations encoded by the hidden space. I am interested in developing tools for learning the geometry of the general space.
Often, the space that the data is sampled from itself is unknown. For example, in computer graphics and machine vision, that space might literally be a geometric object one is trying to learn from imaging data. In another example, in social networks, that space might represent covariates for users with unknown correlations encoded by the hidden space. I am interested in developing tools for learning the geometry of the general space.
Papers
C. Ogle, M. Bevis, D. Asta, J. Fowler, D. Ogle, "On the Upward Continuation Operator and a Conjecture of F. Sanso and M. Sideris," submitted, (2025).
D. Asta, "Lower Bounds for Kernel Density Estimation on Symmetric Spaces," under revision, arXiv preprint 2403.10480, (2024).
D. Asta, "Non-Parametric Manifold Learning," Electronic Journal of Statistics, arXiv preprint 2107.08089, Volume 18(2), pp. 3903-3930, (2024).
C. Shalizi and D. Asta, "Consistency of Maximum Likelihood for Continuous Space Network Models I," Electronic Journal of Statistics, arXiv preprint 1711.02123, Volume 18(1), pp. 335-354, (2023).
D. Asta, A. Davis, T. Krishnamurti, L. Klocke, W. Abdullah, and E. Krans, "The Influence of Social Relationships on Substance Use Behaviors Among Pregnant Women with Opiod Use Disorder," Drug and Alcohol Dependence, Volume 222, (2021).
D. Asta, "Kernel Density Estimation on Symmetric Spaces of Non-Compact Type," Journal of Multivariate Analysis, arXiv preprint 1411.4040, Volume 181, (2021).
A. Smith, D. Asta, and C. Calder, "The Geometry of Continuous Latent Space Models for Network Data," Statistical Science, arXiv preprint 1712.08641, Volume 34(3) pp. 428-453, (2019).
D. Asta, "Kernel Density Estimation on Symmetric Spaces," Proceedings of Geometric Science Information (GSI), Springer LNCS 9398, pages 779-787, (2015).
D. Asta and C. Shalizi, "Geometric Network Comparisons," Proceedings of the 31st Annual Conference on Uncertainty in AI (UAI) [pdf], arXiv preprint 1411.1350, (2015).
D. Asta, "Nonparametric Density Estimation on Hyperbolic Space," Neural Information Processing Systems (NIPS) workshop: Modern Nonparametric Methods in Machine Learning, workshop paper, (2013).
D. Asta and C. Shalizi, "Identifying Influenza Outbreaks via Twitter," Neural Information Processing Systems (NIPS) workshop: Social Network and Social Media Analysis - Methods, Models and Applications, (2012).
D. Asta and C. Shalizi, "Separating Biological and Social Contagions in Social Media: The Case of Regional Flu Trends in Twitter," manuscript in preparation, (2013).
Teaching
I am teaching Probability for Data Analytics (OSU STAT 3201) this Autumn. My office hours are Tuesday and Wednesday 11:00am-12:00pm in Cockins Hall 427. All course material and annoucements are on Carmen.
I've previously taught:
Introduction to Probability for Data Analytics (OSU STAT 3201) in Autumn 2021
Statistical Theory I (OSU STAT 6801) in Autumn 2019
Probability for Statistical Inference (OSU STAT 6301) in Autumn 2016, 2017, 2018, 2020, 2022
Introduction to Time Series (OSU STAT 5550) in Spring 2018, 2019, 2020, 2021, 2022, 2023
Intermediate Data Analysis II (OSU STAT 5302) in Spring 2016, 2017, 2018, 2020, 2021, 2022, 2023
Intermediate Data Analysis I (OSU STAT 5301) (co-taught with James Odei) in Autumn 2015