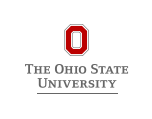
Russell H. Fazio, Ph.D
Harold E. Burtt Professor of Psychology
For an updated version of this website, please see www.russellfazio.com
Site Navigation
BeanFest
BeanFest is a computer game in which participants interact with beans that vary in terms of their shape (from circular to oval to oblong) and number of speckles (from 1 to 10). Different types of beans (e.g., circular beans with few speckles) are associated with either positive or negative outcomes when they are selected. BeanFest was developed by Fazio, Eiser, and Shook (2004) as a paradigm for studying the development of attitudes toward novel objects and the subsequent generalization of those newly-developed attitudes to objects of varying similarity to the original stimuli. The paradigm allows for the assessment of individual differences in the learning of positive versus negative attitudes (what we refer to as the “learning bias”) as well as individual differences in attitude generalization (what we refer to as the valence “weighting bias”). An overview of the program of research is available in a recent Advances in Experimental Social Psychology chapter (Fazio, Pietri, Rocklage, & Shook, 2015).
This webpage is devoted to researchers who may be interested in employing the BeanFest paradigm. Relevant information and resources are noted below.
1. BeanFest Software
We typically run BeanFest via the Inquisit platform. Inquisit is available from http://www.millisecond.com/, and their test library includes some demos and downloadable scripts for running the typical BeanFest procedure, http://www.millisecond.com/download/library/BeanFest/. The script that we ourselves use for running the typical version of BeanFest (points with noncontingent feedback) is available here. This is the implementation we have found most useful for assessing both the learning and the weighting bias. (See the Resources section below for a useful SPSS syntax file specific to the Inquisit version of BeanFest.)
Matt Rocklage has created a Python version of our typical implementation of the BeanFest software. For those familiar with Python, his BeanFestPy program is available here. [A note of caution: this program does not operate properly under a Windows XP platform.] If you have any questions about the python version, feel free to email Matt, Matthew.Rocklage@umb.edu. Also, see the Resources section below for a useful SPSS syntax file that Matt developed for this Python version.
2. Assessing Individual Differences
- The learning bias
- The weighting bias
For any given participant, this equation can be used to compute the predicted value of the participant’s average response to the novel beans, in other words, the value expected on the basis of the individual’s pattern of learning. The difference between the actual value (i.e., the average response computed directly from the participant’s data) and the predicted value serves as the estimate of the given participant’s valence weighting bias.
We would recommend that any researchers using the BeanFest paradigm employ the normative regression equation presented above to compute the predicted values and, ultimately, the residuals that index the weighting bias, provided that their participants can be presumed to be similar to our Ohio State University college student sample. If so, the above regression weights, based as they are on an aggregated sample of over 1800, are likely to prove more stable than those calculated on the basis of a sample-specific regression. If there is reason to question the sample’s similarity to our own student sample, then a regression equation specific to the sample may be more appropriate.
See pp. 108-110 of our recent Advances chapter (Fazio, Pietri, Rocklage, & Shook, 2015) for further details regarding the measurement of valence weighting bias.
3. Recalibrating Individuals’ Valence Weighting Tendencies
In the interest of pursuing questions regarding the causal influence of valence weighting tendencies, we also have developed a recalibration paradigm that modifies an individual’s weighting bias. After learning the positive and negative value of four different types of beans during the initial game phase of BeanFest, participants proceed to the usual test phase in which both previously learned game beans and novel beans that vary in their resemblance to the game beans are presented. The recalibration procedure involves the provision of objectively correct feedback on each and every trial of this test phase. That is, after indicating whether a bean is good or bad, participants are told whether they were correct or not. (See pp. 127-130 of the Advance chapter for further details.) Recalibration essentially operates as an instrumental conditioning procedure, inducing participants toward a more objectively appropriate and balanced weighting of the extent to which a novel bean bears resemblance to a known positive versus a known negative. The end result is that, relative to a control condition in which no feedback is provided, participants who undergo recalibration exhibit a change in their valence weighting tendencies. This change in valence weighting tendencies can first be detected during BeanFest itself: relative to control participants, those in the recalibration condition become significantly more accurate in their classification of novel beans over the course of the test phase. Moreover, this more balanced approach to valence weighting is evident in subsequent situations. Essentially, initially risky individuals become more cautious, and initially cautious become riskier. As such, the recalibration paradigm has proven to be a successful intervention for people with tendencies to overweight negative signals (e.g., those highly sensitive to interpersonal rejection) or overweight positive signals (e.g., those who are highly risky).
As noted earlier, we view the recalibration procedure as an instantiation of instrumental conditioning. We have seen no evidence that participants who undergo recalibration are aware of the changes that they are exhibiting in their valence weighting tendencies, nor that they are pursuing any strategies regarding purposeful correction (see Pietri, Fazio, & Shook, 2013). Additional analyses also have indicated that recalibration does not foster a subsequent tendency to deliberate more extensively (see Fazio, Granados Samayoa, & Pietri, 2020).
4. Resources
This link provides a useful PowerPoint presentation. It is an introductory tutorial describing how to play the BeanFest game. We typically use it to provide initial instructions to the participants.
This link provides a useful SPSS syntax file. After importing the data file created by Inquisit into an SPSS .sav file, the syntax can be used to recode the data. The syntax file ouptuts a new .sav file that includes the BeanFest variables typically of interest. More specifically, the new data file includes variables representing (a) the proportion of positive game beans classified correctly during the test phase, (b) the proportion of negative game beans classified correctly during the test phase, (c) the learning asymmetry, (d) the average response to the novel beans during the test phase, and (e) the valence weighting bias, calculated as described above.
Here is a link to the equivalent SPSS syntax file for the Python version.
4. Bibliography
Publications reporting research stemming from the BeanFest paradigm are listed below in chronological order. Each concerns valence asymmetries in attitude formation and/or attitude generalization, i.e., the learning bias and/or the weighting bias.
Eiser, J. R., Fazio, R. H., Stafford, T., & Prescott, T. J. (2003). Connectionist simulation of attitude learning: Asymmetries in the acquisition of positive and negative evaluations. Personality and Social Psychology Bulletin, 29, 1221-1235.
Fazio, R. H., Eiser, J. R., & Shook, N. J. (2004). Attitude formation through exploration: Valence asymmetries. Journal of Personality and Social Psychology, 87, 293-311.
Shook, N. J., Fazio, R. H., & Vasey, M. W. (2007). Negativity bias in attitude learning: A possible indicator of vulnerability to emotional disorders? Journal of Behavior Therapy and Experimental Psychiatry, 38, 144-155.
Shook, N. J., Fazio, R. H., & Eiser, J. R. (2007). Attitude generalization: Similarity, valence, and extremity. Journal of Experimental Social Psychology, 43, 641-647.
Eiser, J. R., Shook, N. J., & Fazio, R. H. (2007). Attitude learning through exploration: Advice and strategy appraisals. European Journal of Social Psychology, 37, 1046-1056.
Shook, N., Pena, P., Fazio, R. H., Sollers, J. J., & Thayer, J. F. (2007). Friend or foe: Heart rate variability and the negativity bias in learning about novel objects. Psychophysiology, 44, S39 (Abstract).
Deutsch, R., & Fazio, R. H. (2008). How subtyping shapes perception: Predictable exceptions to the rule reduce attention to stereotype-associated dimensions. Journal of Experimental Social Psychology, 44, 1020-1034.
Eiser, J. R., & Fazio, R. H. (2008). How approach and avoidance decisions influence attitude formation and change. In A. J. Elliot (Ed.), Handbook of approach and avoidance motivation (pp. 323-340). New York, NY: Psychology Press.
Eiser, J. R., Stafford, T., & Fazio, R. H. (2008). Expectancy-confirmation in attitude learning: A connectionist account. European Journal of Social Psychology, 38, 1023-1032.
Conklin, L. R., Strunk, D. R., & Fazio, R. H. (2009). Attitude formation in depression: Evidence for deficits in forming positive attitudes Journal of Behavior Therapy and Experimental Psychiatry, 40, 120-126.
Eiser, J. R., Stafford, T., & Fazio, R. H. (2009). Prejudiced learning: A connectionist account. British Journal of Psychology, 100, 399-413.
Shook, N. J., & Fazio, R. H. (2009). Political ideology, exploration of novel stimuli, and attitude formation. Journal of Experimental Social Psychology, 45, 995-998.
Kiken, L.G., & Shook, N.J. (2011). Looking up: Mindfulness increases positive judgments and reduces negativity bias. Social Psychological and Personality Science, 2, 425-431.
Pietri, E. S., Fazio, R. H., & Shook, N. J. (2012). Valence weighting as a predictor of emotional reactivity to a stressful situation. Journal of Social and Clinical Psychology, 31, 746-777.Pietri, E. S., Fazio, R. H., & Shook, N. J. (2013). Weighting positive versus negative: The fundamental nature of valence asymmetry. Journal of Personality, 81, 196-208.
Pietri, E. S., Fazio, R. H., & Shook, N. J. (2013). Recalibrating positive and negative weighting tendencies in attitude generalization. Journal of Experimental Social Psychology, 49, 1100-1113.
Rocklage, M. D., & Fazio, R. H. (2014). Individual differences in valence weighting: When, how, and why they matter. Journal of Experimental Social Psychology, 50, 144-157.
Fazio, R. H., Pietri, E. S., Rocklage, M. D., & Shook, N. J. (2015). Positive versus negative valence: Asymmetries in attitude formation and generalization as fundamental individual differences. In J. M. Olson & M. P. Zanna (Eds.), Advances in Experimental Social Psychology (Vol. 51, pp. 97-146). Burlington: Academic Press.
Pietri, E. S., Vasey, M. W., Grover, M., & Fazio, R. H. (2015). Predicting changes in depressive symptoms from valence weighting during attitude generalization. Journal of Social and Clinical Psychology, 34, 859-875.
Pietri, E. S., & Fazio, R. H. (2017). Recalibrating valence weighting biases to promote changes in rejection sensitivity and risk-taking. Journal of Experimental Social Psychology, 68, 1-10.
Zunick, P. V., Granados Samayoa, J. A., & Fazio, R. H. (2017). The role of valence weighting in impulse control. Journal of Experimental Social Psychology, 72, 32-38.
Rocklage, M. D., Pietri, E. S., & Fazio, R. H. (2017). The weighting of positive vs. negative valence and its impact on the formation of social relationships. Journal of Experimental Social Psychology, 73, 65-75.
Pietri, E. S., Dovidio, J. F., & Fazio, R. H. (2018). Recalibrating valence weighting tendencies as a means of reducing anticipated discomfort with an interracial interaction. Group Processes & Intergroup Relations, 21, 597-614.
Niese, Z. A., Libby, L. K., Fazio, R. H., Eibach, R. P., & Pietri, E. S. (2019). Does the future look bright? Processing style determines the impact of valence weighting biases and self-beliefs on expectations. Journal of Personality and Social Psychology, 116, 193-214.
Heylen, J., De Raedt, R., Rocklage, M. D., Fazio, R. H., Vasey, M. W., & Bosmans, G. (2019). From trust in caregivers’ support to exploration: The role of openness to negative affect and self-regulation. Scandinavian Journal of Psychology, 60, 309-322.
Ram, H., & Liberman, N. (2019). The relative contribution of response bias and weighting-of-similarity bias to valence asymmetry in attitude generalization. Journal of Experimental Social Psychology, 85.
Granados Samayoa, J. A., & Fazio, R. H. (2021). “I want it now!” Intertemporal choice through the lens of valence weighting bias. Social Cognition, 39, 243–258.